Получена: 07.04.2023/ Принята: 20.05.2023/ Опубликована online: 30.06.2023
УДК 616.896-08
DOI 10.53511/PHARMKAZ.2023.32.31.007
Кошербаева Ляззат1, Самамбаева Айжан2, Сабырділда Жанар1, Кожагельдиева Лаура3
1 НАО «Казахский национальный медицинский университет имени С. Д. Асфендиярова», Алматы, Казахстан
2 AYeconomics Research Centre S.L., Сантьяго де Компостела, Испания
3 Университет Сулеймана Демиреля, Алматы, Казахстан
РОЛЬ ИСКУССТВЕННОГО ИНТЕЛЛЕКТА ДЛЯ ЛЕЧЕНИЯ ДЕТЕЙ С РАССТРОЙСТВОМ АУТИСТИЧЕСКОГО СПЕКТРА: МЕЖДУНАРОДНЫЙ ОПЫТ
Резюме: Введение. Расстройства аутистического спектра (РАС) — это расстройство развития
нервной системы, для которых характерны поведенческие и психологические проблемы у детей.
Наблюдается рост распространённости РАС во всем мире. В последние десятилетия наблюдается
рост исследований по применению искусственного интеллекта (ИИ), позволяющий ускорить процесс диагностики, и впоследствии раннего доступа к лечению детей с РАС.
Целью нашего обзора является изучить применение искусственного интеллекта для детей с РАС.
Методы. Был проведен обзор литературы существующих систематических обзоров и мета-анализов с 2013 по 2023 г. Поиск литературы проводился в базах данных PubMed, Кокрановская база систематических обзоров и Scopus. Поиск был ограничен человеческой популяцией, исследованиями на
английском и русском языке. Каждое включенное исследование оценивалось по чек-листу AMSTAR-2.
Результаты. Мы проанализировали результаты пяти систематических обзоров, двух мета-анализов, трех систематических обзоров-мета-анализов. В настоящее время нет единых подходов применения ИИ для диагностики и лечения детей с РАС. Существующие алгоритмы машинного обучения подразделяется на контролируемое, полу-контролируемое, неконтролируемое. Большинство работ включали в себя оценку производительности машины опорных векторов, нейронные сети и деревья решений, из которых наибольшую эффективность продемонстрировал анализ нейронной сети.
ИИ применяется различными способами для диагностики и лечения РАС. Важно отметить, что
системы на основе ИИ все еще разрабатываются и проверяются, и не все они еще широко применяются в клинической практике. Кроме того, модели ИИ следует использовать как дополнительный инструмент, а не как единственный метод диагностики, и всегда под наблюдением квалифицированного специалиста.
Выводы. Проведенный анализ показал рост исследований в области ИИ для диагностики и лечения
РАС, который имеет большой потенциал в будущих разработках и производительности.
Ключевые слова: дети с расстройством аутистического спектра; искусственный интеллект;
диагностика; машинное обучение
СПИСОК ЛИТЕРАТУРЫ
1 American Psychiatric Association. Diagnostic and statistical manual of mental disorders. 5th ed. Arlington: American Psychiatric Publishing, 2013.
2 World Health Organization (2019). Autism spectrum disorders. Available at: https://www.who.int/news-room/fact-sheets/detail/autism-spectrum-disorders
3 Fombonne E (2009) Epidemiology of pervasive developmental disorders. Pediatr Res 65:591–598 72. Halladay AK, Bishop S, Constantino JN, Daniels AM,
Koenig K, Palmer K, Messinger D, Pelphrey K, Sanders SJ, Singer AT, Taylor JL, Szatmari P (2015) Sex and gender diferences in autism spectrum disorder:
summarizing evidence gaps and identifying emerging areas of priority. Molecular autism 6:36
4 Bougeard C, Picarel-Blanchot F, Schmid R, Campbell R, Buitelaar J. Prevalence of Autism Spectrum Disorder and Co-morbidities in Children and Adolescents:
A Systematic Literature Review. Front Psychiatry. 2021 Oct 27;12:744709. doi: 10.3389/fpsyt.2021.744709.
5 Baio J, Wiggins L, Christensen DL, Maenner MJ, Daniels J, Warren Z, et al. Prevalence of autism spectrum disorder among children aged 8 years — autism
and developmental disabilities monitoring network, 11 sites, United States, 2014. MMWR Surveill Summ. 2018;67:1.
6 Qiu S, Lu Y, Li Y, Shi J, Cui H, Gu Y, et al. Prevalence of autism spectrum disorder in Asia: A systematic review and meta-analysis. Psychiatry Res.
2020;284:112679.
7 Delobel-Ayoub M, Ehlinger V, Klapouszczak D, Maffre T, Raynaud JP, Delpierre C, et al. Socioeconomic disparities and prevalence of autism spectrum
disorders and intellectual disability. PLoS One. 2015;10(11):e0141964.
8 Davidovitch M, Hemo B, Manning-Courtney P, Fombonne E. Prevalence and incidence of autism spectrum disorder in an Israeli population. J Autism Dev
Disord. 2013;43:785–793.
9 Alshaban F, Aldosari M, Al-Shammari H, El-Hag S, Ghazal I, Tolefat M, et al. Prevalence and correlates of autism spectrum disorder in Qatar: a national study.
J Child Psychol Psychiatry Allied Discip. 2019;60:1254–1268
10 Mohammadi MR, Ahmadi N, Khaleghi A, Zarafshan H, Mostafavi SA, Kamali K, et al. Prevalence of autism and its comorbidities and the relationship with
maternal psychopathology: a national population-based Study. Arch Iran Med. 2019;22:546–553.
11 Yoo SM, Kim KN, Kang S, Kim HJ, Yun J, Lee JY. Prevalence and Premature Mortality Statistics of Autism Spectrum Disorder Among Children in Korea: A
Nationwide Population-Based Birth Cohort Study. J Korean Med Sci. 2022 Jan 3;37(1):e1. doi: 10.3346/jkms.2022.37.e1.
12 Zhou H, Xu X, Yan W, Zou X, Wu L, Luo X, Li T, Huang Y, Guan H, Chen X, Mao M, Xia K, Zhang L, Li E, Ge X, Zhang L, Li C, Zhang X, Zhou Y, Ding D,
Shih A, Fombonne E, Zheng Y, Han J, Sun Z, Jiang YH, Wang Y; LATENT-NHC Study Team. Prevalence of Autism Spectrum Disorder in China: A Nationwide
Multi-center Population-based Study Among Children Aged 6 to 12 Years. Neurosci Bull. 2020 Sep;36(9):961-971. doi: 10.1007/s12264-020-00530-6. Epub
2020 Jun 30.
13 Smith DaWalt L, Hong J, Greenberg JS, Mailick MR. Mortality in individuals with autism spectrum disorder: Predictors over a 20-year period. Autism. 2019
Oct;23(7):1732-1739. doi: 10.1177/1362361319827412. Epub 2019 Feb 28.
14 Sharma M. Improved autistic spectrum disorder estimation using Cfs subset with greedy stepwise feature selection technique. International Journal of
Information Technology . 2022;14(3):1251–1261. doi: 10.1007/s41870-019-00335-5
15 Sobieski M, Sobieska A, Sekułowicz M, Bujnowska-Fedak MM. Tools for early screening of autism spectrum disorders in primary health care — a scoping
review. BMC Prim Care. 2022 Mar 15;23(1):46. doi: 10.1186/s12875-022-01645-7.
16 Moridian P, Ghassemi N, Jafari M, Salloum-Asfar S, Sadeghi D, Khodatars M, Shoeibi A, Khosravi A, Ling SH, Subasi A, Alizadehsani R, Gorriz JM, Abdulla
SA, Acharya UR. Automatic autism spectrum disorder detection using artificial intelligence methods with MRI neuroimaging: A review. Front Mol Neurosci. 2022
Oct 4;15:999605. doi: 10.3389/fnmol.2022.999605.
17 Keele, S. Guidelines for Performing Systematic Literature Reviews in Software Engineering; Technical Report Ver. 2.3; EBSE: Durham, UK, 2007
18 Shea BJ, Reeves BC, Wells G, Thuku M, Hamel C, Moran J, Moher D, Tugwell P, Welch V, Kristjansson E, Henry DA. AMSTAR 2: a critical appraisal tool
for systematic reviews that include randomised or non-randomised studies of healthcare interventions, or both. BMJ. 2017 Sep 21;358:j4008.
19 Zhang S, Wang S, Liu R, Dong H, Zhang X, Tai X. A bibliometric analysis of research trends of artificial intelligence in the treatment of autistic spectrum
disorders. Front Psychiatry. 2022 Aug 29;13:967074. doi: 10.3389/fpsyt.2022.967074.
20 Minissi ME, Chicchi Giglioli IA, Mantovani F, Alcañiz Raya M. Assessment of the Autism Spectrum Disorder Based on Machine Learning and Social Visual
Attention: A Systematic Review. J Autism Dev Disord. 2022 May;52(5):2187-2202. doi: 10.1007/s10803-021-05106-5. Epub 2021 Jun 8.
21 Cano S, González CS, Gil-Iranzo RM, Albiol-Pérez S. Affective Communication for Socially Assistive Robots (SARs) for Children with Autism Spectrum
Disorder: A Systematic Review. Sensors (Basel). 2021 Jul 30;21(15):5166. doi: 10.3390/s21155166.
22 Alqaysi ME, Albahri AS, Hamid RA. Diagnosis-Based Hybridization of Multimedical Tests and Sociodemographic Characteristics of Autism Spectrum Disorder
Using Artificial Intelligence and Machine Learning Techniques: A Systematic Review. Int J Telemed Appl. 2022 Jul 1;2022:3551528. doi: 10.1155/2022/3551528.
23 Raj S., Masood S. Analysis and detection of autism spectrum disorder using machine learning techniques. Procedia Comput. Sci. 2020;167:994–1004.
doi: 10.1016/j.procs.2020.03.399.
24 Stevens E., Dixon D. R., Novack M. N., Granpeesheh D., Smith T., Linstead E. Identification and analysis of behavioral phenotypes in autism spectrum
disorder via unsupervised machine learning. International Journal of Medical Informatics . 2019;129(August):p. 29. doi: 10.1016/j.ijmedinf.2019.05.006
25 Peral J., Gil D., Rotbei S., Amador S., Guerrero M., Moradi H. A machine learning and integration based architecture for cognitive disorder detection used
for early autism screening. Electronics . 2020;9(3) doi: 10.3390/electronics9030516.
26 Misman M. F., Samah A. A., Ezudin F. A., et al. Proceedings — 2019 1st International Conference on Artificial Intelligence and Data Sciences . AiDAS 2019;
2019. Classification of adults with autism spectrum disorder using deep neural network; pp. 29–34
27 Hassan M. M., Mokhtar H. M. O. Investigating autism etiology and heterogeneity by decision tree algorithm. Informatics Med. Unlocked . 2019;16, article
100215 doi: 10.1016/j.imu.2019.100215.
28 Tyagi B., Mishra R., Bajpai N. Machine learning techniques to predict autism spectrum disorder; 1st International Conference on Data Science and Analytics,
PuneCon 2018 — Proceedings ; 2018. pp. 1–5.
29 Lawi A., Aziz F. Proceedings — 2nd East Indonesia Conference on Computer and Information Technology: Internet of Things for Industry . EIConCIT 2018;
2018. Comparison of classification algorithms of the autism Spectrum disorder diagnosis; pp. 218–222.
30 Ogunleye A., Wang Q. G., Marwala T. Integrated learning via randomized forests and localized regression with application to medical diagnosis. IEEE Access
. 2019;7:18727–18733. doi: 10.1109/ACCESS.2019.2893349.
31 Cavus N, Lawan AA, Ibrahim Z, Dahiru A, Tahir S, Abdulrazak UI, Hussaini A. A Systematic Literature Review on the Application of Machine-Learning Models
in Behavioral Assessment of Autism Spectrum Disorder. J Pers Med. 2021 Apr 14;11(4):299. doi: 10.3390/jpm11040299.
32 Gautam R, Sharma M. Prevalence and Diagnosis of Neurological Disorders Using Different Deep Learning Techniques: A Meta-Analysis. J Med Syst. 2020
Jan 4;44(2):49. doi: 10.1007/s10916-019-1519-7.
33 Li, G., Liu, M., Sun, Q., Shen, D., and Wang, L., Early diagnosis of autism disease by multi-channel CNNs. In International Workshop on Machine Learning
in Medical Imaging, Springer, Cham,303–309, 2018.
34 Guo, X., Dominick, K. C., Minai, A. A., Li, H., Erickson, C. A., and Lu, L. J., Diagnosing autism spectrum disorder from brain resting-state functional
connectivity patterns using a deep neural network with a novel feature selection method. Front Neurosci 11(1–19):460, 2017
35 Kong, Y., Gao, J., Xu, Y., Pan, Y., Wang, J., and Liu, J., Classification of autism spectrum disorder by combining brain connectivity and deep neural network
classifier. Neurocomputing 324:63–68, 2019.
36 Rad, N. M., and Furlanello, C., Applying deep learning to stereotypical motor movement detection in autism spectrum disorders. In 2016 IEEE 16th
International Conference on Data Mining Workshops IEEE, 1235–1242, 2016.
37 Heinsfeld, A. S., Franco, A. R., Craddock, R. C., Buchweitz, A., and Meneguzzi, F., Identification of autism spectrum disorder using deep learning and the
ABIDE dataset. NeuroImage: Clinical 17:16–23, 2018.
38 Song DY, Topriceanu CC, Ilie-Ablachim DC, Kinali M, Bisdas S. Machine learning with neuroimaging data to identify autism spectrum disorder: a systematic
review and meta-analysis. Neuroradiology. 2021 Dec;63(12):2057-2072. doi: 10.1007/s00234-021-02774-z. Epub 2021 Aug 22.
39 Barttfeld P, Wicker B, Cukier S, Navarta S, Lew S, Sigman M (2011) A big-world network in ASD: dynamical connectivity analysis refects a defcit in longrange connections and an excess of short-range connections. Neuropsychologia 49:254–263
40 Santana CP, de Carvalho EA, Rodrigues ID, Bastos GS, de Souza AD, de Brito LL. rs-fMRI and machine learning for ASD diagnosis: a systematic review
and meta-analysis. Sci Rep. 2022 Apr 11;12(1):6030. doi: 10.1038/s41598-022-09821-6
41 Quaak M, van de Mortel L, Thomas RM, van Wingen G. Deep learning applications for the classification of psychiatric disorders using neuroimaging data:
Systematic review and meta-analysis. Neuroimage Clin. 2021;30:102584. doi: 10.1016/j.nicl.2021.102584
42 Yücelbaş Ş., Yücelbaş C. Autism spectrum disorder detection using sequential minimal optimization-support vector machine hybrid classifier according to
history of jaundice and family autism in children. Concurrency Computat Pract Exper . 2022;34(1) doi: 10.1002/cpe.6498.
43 Koprowski R, Foster KR (2018) Machine learning and medicine: book review and commentary. Biomed Eng Online 17:17
44 Uddin S, Khan A, Hossain ME, Moni MA (2019) Comparing diferent supervised machine learning algorithms for disease prediction. BMC Med Inform Decis
Mak 19:281
45 Tomasiello S (2020) A granular functional network classifer for brain diseases analysis. Comput Methods Biomech Biomed Engin 8:382–388
46 Xiao X, Fang H, Wu J, Xiao C, Xiao T, Qian L, Liang F, Xiao Z, Chu KK, Ke X (2017) Diagnostic model generated by MRIderived brain features in toddlers
with autism spectrum disorder. Autism Res 10:620–630
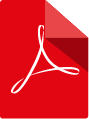